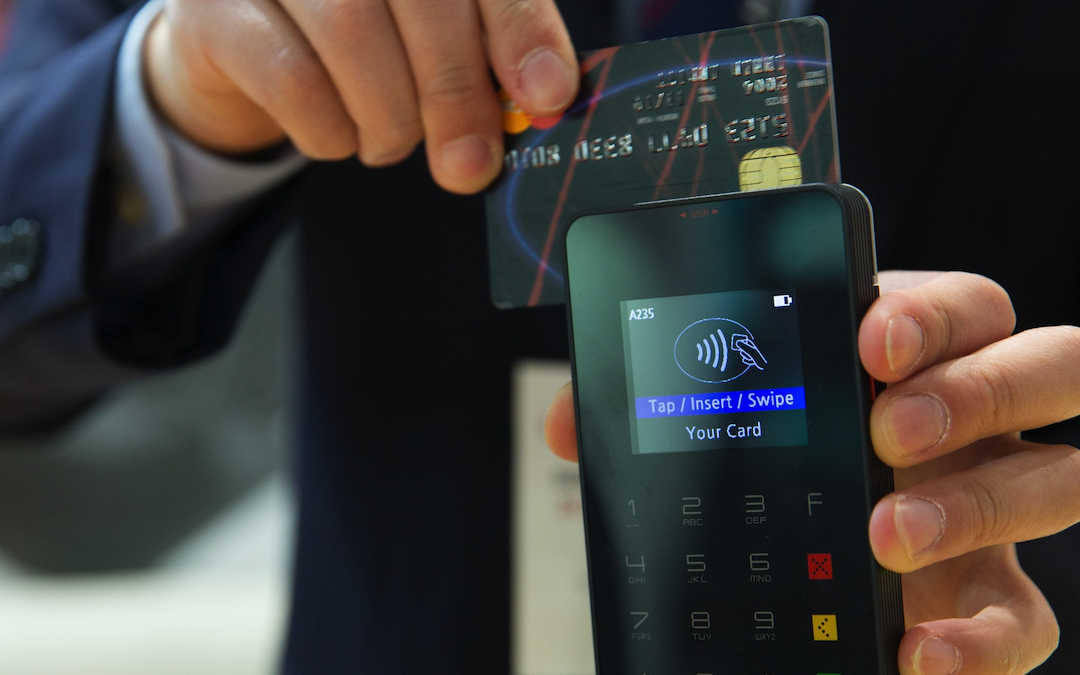
The intersection of artificial intelligence (AI) and machine learning (ML) with the financial technology sector (fintech) has ushered in a new era of risk management. As fintech trends continue to evolve, these technologies play a pivotal role in enhancing risk assessment, fraud detection, and operational efficiency. In this article, we delve into the lesser-explored aspects of AI and ML in fintech risk management.
The financial landscape is undergoing rapid transformation, driven by technological advancements. Fintech companies are leveraging AI and ML to revolutionize risk management practices. Beyond the buzzwords, let’s explore how these innovations are reshaping the industry.
Understanding Risk Management in Fintech
Risk management in fintech extends beyond credit risk and market volatility. It encompasses cybersecurity, regulatory compliance, and operational resilience. Fintech startups often operate in dynamic environments, where traditional risk models fall short. AI-powered algorithms can analyze vast datasets, identify patterns, and predict risks more accurately. By considering non-traditional data sources (such as social media activity or transaction histories), fintech companies gain deeper insights into customer behavior and potential risks.
AI and Machine Learning: Transforming Risk Assessment
Predictive Modeling Beyond the Obvious
AI-driven predictive models go beyond linear regression. They explore complex relationships, uncover hidden correlations, and adapt to changing market conditions. For instance, ML algorithms can predict credit defaults by analyzing not only financial data but also behavioral cues. These models continuously learn from new data, improving their accuracy over time.
Anomaly Detection: The Silent Guardian
Anomalies—fraudulent transactions, irregular trading patterns, or sudden market shifts—are often subtle. AI excels at anomaly detection, flagging suspicious activities that human analysts might miss. By combining historical data with real-time monitoring, fintech systems can swiftly identify deviations from the norm.
Automated Fraud Detection and Prevention
Behavioral Biometrics
Fintech trends now embrace behavioral biometrics—analyzing user interactions (keystrokes, mouse movements) to verify identity. Unlike static credentials, these dynamic markers adapt to user behavior. AI algorithms recognize anomalies, such as a sudden change in typing speed, and trigger alerts for potential fraud.
Unsupervised Learning for Fraud Patterns
Traditional rule-based fraud detection systems struggle with emerging fraud schemes. Unsupervised ML techniques, like clustering and autoencoders, learn from unlabeled data. They detect novel patterns without predefined rules, making them adept at catching evolving fraud tactics.
Enhancing Credit Scoring Models
Beyond Credit Histories
AI augments credit scoring by incorporating unconventional data sources. Social media profiles, e-commerce behavior, and even smartphone usage patterns provide valuable insights. Fintech lenders can assess creditworthiness more holistically, especially for thin-file or no-file applicants.
Explainable AI for Transparency
As AI models become more complex, transparency becomes crucial. Explainable AI techniques—such as SHAP (SHapley Additive exPlanations)—help fintech professionals understand model decisions. Balancing predictive power with interpretability ensures fair lending practices.
Market Risk Prediction and Portfolio Optimization
Sentiment Analysis and Market Sentiment
AI analyzes news articles, social media sentiment, and investor sentiment to predict market movements. By quantifying emotions, fintech firms gain an edge in portfolio management. ML algorithms optimize asset allocation, considering risk appetite and market dynamics.
Robo-Advisors: AI-Driven Investment Guidance
Robo-advisors blend AI with human expertise. They recommend personalized investment strategies based on risk tolerance, financial goals, and market conditions. These automated advisors democratize wealth management, catering to a broader audience.
Operational Risk Mitigation with AI
NLP for Contract Analysis
Natural language processing (NLP) automates contract review, ensuring compliance and minimizing legal risks. AI parses complex agreements, identifies clauses, and alerts stakeholders to potential pitfalls.
Process Automation and Error Reduction
AI streamlines operational workflows, reducing manual errors. From KYC (Know Your Customer) processes to trade settlements, automation enhances efficiency and reduces operational risks.
Challenges and Ethical Considerations
Bias Mitigation
AI models inherent biases from training data. Fintech professionals must actively address bias to ensure fair outcomes. Regular audits, diverse datasets, and ethical guidelines are essential.
Regulatory Compliance
As fintech embraces AI, regulatory bodies grapple with oversight. Striking a balance between innovation and compliance is critical. Collaborative efforts are needed to shape responsible AI policies.
Conclusion
Fintech trends converge with AI and ML, reshaping risk management paradigms. By embracing transparency, innovation, and ethical practices, the industry can harness the full potential of these technologies. As fintech continues to evolve, risk management will remain at the forefront of transformation.
Share this post
Leave a comment
All comments are moderated. Spammy and bot submitted comments are deleted. Please submit the comments that are helpful to others, and we'll approve your comments. A comment that includes outbound link will only be approved if the content is relevant to the topic, and has some value to our readers.
Comments (0)
No comment